An introduction to AIOps and how it can be utilized in ITIL® 4
White Paper
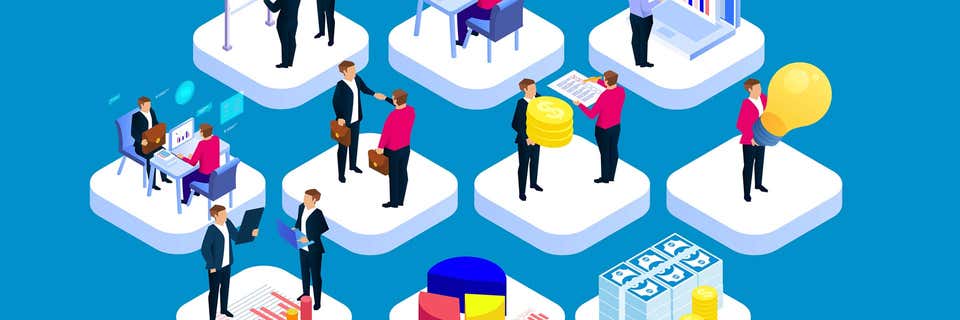
White Paper
- White Paper
- Business solutions
- Collaboration
- DevOps
- Digital transformation
- IT Services
- ITIL
May 26, 2020 |
24 min read
- White Paper
- Business solutions
- Collaboration
- DevOps
- Digital transformation
- IT Services
- ITIL
The digital transformation has led to a paradigm shift for how IT services are designed, developed, delivered, and operated. Organizations all over the world are exploring new emerging technologies and agile ways of working. ITIL 4 has evolved to help organizations adapt modern technologies and new working methods; it is designed to collaborate with many frameworks and methods within the IT industry. As one of these methods, AIOps is an important area for organizations to explore, to enhance their service management capabilities, and prepare for future ways of delivering IT services.
Artificial Intelligence (AI) Used to describe machines that mimic cognitive functions, which are normally associated with the human mind, such as learning and problem solving. Machine learning The scientific study of the algorithms and statistical models used by computer systems to perform a specific task, without using explicit instructions, and relying on patterns. It is seen as a subset of artificial intelligence. IT analytics The use of mathematical algorithms and other innovations to extract meaningful information from the collection of raw data gathered by management and monitoring technologies. Artificial intelligence for IT operations (AIOps) Technology that enhances IT analytics using big data analytics, machine learning, and other AI technologies to automate the identification and resolution of common IT issues. AIOps functionality AIOps functionality added to existing tools, for example, applying big data and machine learning in integrated service management tools to analyze the effectiveness of a service desk. AIOps platforms A big data platform that can collect IT operations data from various sources and use advanced machine learning to support all primary IT operations functions The AI effect As machines become increasingly sophisticated, technology that was considered highly advanced become commonplace. For example, optical character recognition is no longer seen as AI. |
1.1 A BRIEF HISTORY OF AI AND MACHINE LEARNING
Artificial intelligence (AI) is the practice of enabling machines to perform tasks that are normally associated with the human brain. Some tasks are performed better by machines than by humans, such as processing a large amount of data to recognize patterns. However, humans still need to communicate their objectives to the machine. An important area of AI and machine learning is to teach the machine how to: understand patterns, conclude, and implement the results when the calculation is complete. It is about creating algorithms to allow a machine to recognize, analyse, and solve complicated challenges.One area where AI has been used extensively is within the digital entertainment sector. As early as 1997, a machine was able to beat the world chess champion. Yet, chess is a straightforward game compared to the Chinese board game GO. As one of the world’s most advanced board games, requiring high-level strategic thinking and problem-solving, it was for a long time seen as impossible for a machine to master.
However, great minds continued to improve AI technology. In October 2015, the Google computer program AlphaGo became the first computer to beat a human professional GO player without handicaps. It required a new generation of machine learning, feeding the machine enormous amounts of training data and algorithms, resulting in strategies that allow the computer to explore problems, test solutions, and learn from its failures. When AlphaGo beat the world champion Ke Jie in a five-game match in May 2017, the Chinese world champion burst into tears. Afterwards, it was said that the result was not a failure of Ke Jie, but a failure of mankind. On the other hand, it not only proved the success of AI, but also the success of mankind who had created the AI.
The exploration and use of AI and machine learning has developed exponentially with the emergence of new technologies such as big data, the internet of things (IoT), and cloud computing. The emergence of big data and AI is tightly linked. What is the point of gathering data, if the data is not processed, analysed, interpreted and fulfilled? AI also justifies the existence of certain new technologies.
The development of AI and machine learning over the years has enabled a new generation of services. For example, facial recognition and fingerprint scanning has become a part of normal life. IoT and robotics have been introduced in homes in the form of tools like vacuum cleaners and lawn mowers. Advanced monitoring, combined with machine learning and automated decision-making, has even produced self-driving cars, which are said to be more reliable than human drivers.
Many organizations are exploring how AI can be used to establish new services and realize business goals. It is, for example, used to predict business activities and sales. In this paper we will focus on how AI can be utilized within IT operations. This will be referred to as AIOps.
What is AIOps and how does it work?
It has become a challenge for IT operations to manage the growing volume of data that should be captured and analysed. Problem analysis can be a difficult and time-consuming task, especially since traditionally it has been the responsibility of siloed teams to monitor their workload with different tools.
The term AIOps (algorithmic operations) was introduced by Gartner in 2017 and refers to big data, analytics, and machine learning used to help IT operations identify and resolve high priority incidents faster and detect potential problems before incidents happen.
Instead of siloed teams looking at their own logfiles, AIOps explores how important data can be gathered in one place, to allow the machine to process data from different sources and utilize AI and machine learning to recognize problems. It can be used to extract information from the pool of operational data, foresee and avoid interruptions, and provide knowledge on how IT services are used.
Essentially, AIOps can help IT operations with three things:
- Automate routine tasks so that the IT operations teams can focus on more strategic work.
- Perform tasks beyond human capabilities, such as:
- data processing to detect patterns or abnormities
- analysing these abnormities, identifying causes.
- Taking the right action, based on the causes found and conclusions drawn.
The difference between AIOps platforms and AIOps functionality
In this paper we will describe two different concepts within AIOps: AIOps platforms and AIOps functionality.
AIOps functionality AI and machine learning can be utilized within specific domains, for example to analyse data from integrated service management tools. Exploring AIOps functionality within smaller areas is a great way for operational staff to explore how AI can be helpful.
AIOps platforms AI Ops platforms is taking it a big step further, using big data technology to collect operational data from different sources. This is to utilize machine learning and other advanced analytics technologies and enhance IT operations functions with proactive, personal, and dynamic insight. The purpose of the AIOps platform is to gather the generated data in one place, enabling the concurrent use of multiple data sources, data collection methods, analytical technologies, and presentation technologies. It is still in the early phase of development. Gartner expects that the use of big data platforms for operations will increase from 5% in 2018 to 30% in 20231.
Even if there are systems on the market that are ready for AIOps platforms, it might take some time for organizations to utilize the new technology. The vendors know the technology and organizations know its data. Vendors and organizations will therefore need to work together to explore how to build the AIOps platform and how to grow the maturity of the platform step by step. This is shown in Table 1.1
Expectations of an AIOps platform (The service provider/vendor responsibility) |
How to utilize an AIOps platform (The service consumer responsibility) |
---|---|
The technology should include data collection methods and concurrent use of multiple data sources. It should be able to gather both historical data and streaming data from logfiles, key metrics, and SLA targets. | IT operations need to clearly define the problems that they want to be resolved with AI and provide the necessary data. |
Most organizations have an overwhelming number of different tools. A true AIOps platform will therefore have to use event correlation analysis to reduce duplication and irrelevant information. | IT operations should identify the right data sources and check the coverage and quality of the data. |
The platform should include machine learning functionality, which will allow the system to recognize patterns and anomalies. | The IT operations team should have enough knowledge about statistics and analytics to be able to understand how different algorithms work. |
It should be able to conduct problem analysis and automated actions based on the conclusions. | At the start, the IT operation team might complete the necessary actions manually. They may become more familiar with the system and allow more machine learning and automated actions. |
Key message One of the most important skills to develop in IT operations will be the ability to identify real issues. Then you can explore how AI and machine learning can help. |
THE IMPORTANCE OF RELIABLE DATA IN MACHINE LEARNING
Although the AIOps platform can collect and organize data, the data inputted must be of sufficient quality and accurate. Machine learning requires a large data set for training. Both historical and real-time data can be used to train the machine to recognize patterns and provide responses. However, the data set needs to be of sufficient size, quality, and representative of the overall outputs. Otherwise, there is a risk that the machine could be trained to make the wrong decision.
The importance of this can be illustrated with a story about Tay, launched in March 2016. Tay was a Twitter bot described as an experiment in conversational understanding. The intention was to teach Tay to engage with people through ‘casual and playful conversation.’ Unfortunately, the conversations did not stay playful for long. Soon after Tay was launched, people started tweeting the bot with all sorts of ugly and impolite words. Tay, which was essentially a robot parrot, started to repeat these words back to users. By the end of the day, Tay had become so rude that they had to close her down!
Key message With machine learning, remember: garbage in creates garbage out. |
How to start with AIOps
The continual improvement model can be a guide on how to start with AIOps.
Activity | Examples of continual improvement |
---|---|
What is the vision? | What is the overall objective? What types of services does the organization deliver and support? Who are the customers? |
Where are we now? | How is the current situation? How happy are the customers? Where are the pain points? |
Where do we want to be? | What could be a suitable test case for AIOps? Where could it provide most value? What areas do we need to standardize and automate? |
Take action | Take the small step approach. Choose a test case. Test it out, see how it works and learn from it |
Did we get there? | When starting with AIOPs, this step should be an integral part of the two previous ones, a continuous loop of plan, do, check, and act in small increments |
How to keep momentum going? | Key learnings from the test case to be captured in the next project |
Table 3.1 Continual improvement model on how IT operations can use AIOps
CHOOSING THE INITIAL TEST CASE
AIOps should create value. A good test case for AIOps should be as specific as possible, to provide the most value and represent an area where the IT operations team needs to improve. Since IT operations is there to support the business, the effect on the business should also be considered.
Some examples could include:
- One specific value stream of the business: how can the team monitor every operational step in the process, the overall performance of the service, and the perceived user experience?
- The pipeline of a DevOps team: how the team create a self-help platform for itself, which monitors all the steps, and automates the full pipeline?
- A self-help portal at the service desk: how to use AI and machine learning to provide solutions faster, and at the same time provide a great user experience?
CHOOSE AN INCREMENTAL APPROACH
Organizations should explore the field of AIOps as soon as possible. It is recommended to use an incremental approach in the adaption of AIOps. AIOps functionality can be explored within existing tools. This will allow the IT operations teams to gain experience in how AI works and start building the analytical skills needed to use AI efficiently.
The process for evolving AIOps platforms will typically go through three different stages:
- Monitor: recognize patterns in descriptive data
- Learn: perform anomaly detection and diagnostics
- Build: when the learn stage is completed, it can then perform proactive operations and be able to use all of this to help avoid high-severity outages entirely.
This will require continual improvement of the IT operations maturity, including monitoring and data quality, as well as a constant development of knowledge and experience within the operation team.
BREAKING DOWN SILOS
Historically, IT staff have been organized vertically based on the technology stack they managed. The emergence of AIOps platforms will require collaboration across teams, a new culture, and a new mindset for the people within IT operations. They will need to understand the value streams and use cross functional competence to explore how AIOps can support it.
KNOWLEDGE, SKILLS, AND MINDSET
In the future, the key skills needed within IT operations will lean towards a more standardized, cloud-based platform services with a high degree of monitoring and automation. The operations team will need to acquire new knowledge and skills as highlighted in Table 3.2. It may also require a special mindset to master AIOps.
Knowledge , skills and mindset | Description |
---|---|
An engineering mindset | The ability to exploit new tools to solve particular problems. When a routine task is identified, they might use scripting to optimize and automate the task. |
Business understanding | The ability to ask the right questions to identify problems and needs that can be supported by AIOps. |
Analytical skills | The ability to identify hidden answers in data, gathering the necessary data, and processing the right data using algorithms. This also includes using the appropriate actions when different abnormalities are detected. |
Statistics and analytics | IT operations should not treat AI and machine learning as black boxes that effortlessly provide solutions. They need to establish enough knowledge about statistics to be able to understand, trust, and utilize advanced machine learning solutions provided by specialized vendors. |
AIOps tool knowledge | Choose a few tools and experiment on how to use them. Remember to utilize the specialized competence of the vendor. |
Continual improvement | AIOps will require people to work together across silos. AIOps is an advanced field, and people must develop their experience and continuously seek feedback for the value created. |
Table 3.2 Knowledge, skills, and mindset needed to master AIOps
Areas where AIOps is already in use
There are several areas where AIOps is already in use (and already was when the term AIOps was introduced). In the following section we will look at how the concept of AIOps platforms are used within DevOps. We will also give some examples of how AIOps functions have been used within ITIL practices. Some organizations have even started to use AIOps in their business communications.
AIOPS WITHIN DEVOPS
The purpose of DevOps is to make development and operations part of the same value stream. As an alternative to the complexity of traditional IT, the introduction of cloud computing contributed greatly to the success of DevOps. Standardized environments provided as platform as a service (PaaS), together with the invention of handling Infrastructure as a Code (IaaC), led to a greater development of the DevOps movement. Another enabler was the introduction of microservices and containerization, that has made it possible to deploy smaller, single-function modules, without a risk of affecting the entire application.
With a mindset of ‘automate everything you can’, software features are now being deployed directly into live environments at a rate that is both safer and faster than ever before. Extensive use of AIOps has allowed for automated actions including automated provisioning, automated integration, automated testing and deployment. Each step is subject to extensive monitoring to provide fast feedback. Without the use of machine learning and automated actions, the excessive amount of metering and monitoring data would be impossible to use.
Figure 4.1 AIOps as an enabler in a DevOps environment
AIOPS WITHIN SERVICE MANAGEMENT PRACTICES
AIOps has been used in various ways in IT and service management. It is a tool that can help many practices support value chain activities, as described in further detail below.
Monitoring and event management
- A common challenge when using monitoring tools to manage large IT infrastructure environments is separating the signal from noise. Automatic noise detection can ensure that the noise is filtered, and only relevant and important events are suggested for analysis by human specialists.
- AIOps can also use a variety of supervised or unsupervised techniques to collate related alerts together into a single incident record, to avoid duplicate tickets.
Incident management
- AIOps functionality within service management tools is already able to analyse historical data and highlight areas of concern. It might also allow automated classification of incidents/service requests.
- It might also help detect and correct incidents before it is visible to the user.
- For swarming (a technique of specialists from different areas working together to solve problems), an AIOps platform with data from many sources would save time and help gain a common understanding.
Capacity management
- Monitoring capacity could trigger a script to automatically provide additional capacity. It could also automatically detach capacity when it is no longer needed.
- AIOps could also be used to analyse actual usage of services by the organization, its users and customers, identify patterns of business activity (PBA), and establish proactive actions based on this.
Deployment management
- In a cloud environment, AIOps could help choose the right type of virtual machine for provisioning.
- In DevOps, AIOps is used to automate the full deployment pipeline.
Information security
- In addition to pattern recognition, a common function of AIOPs is anomaly detection. This uses the patterns discovered by the previous steps to determine normal system behaviour, and then react when it discovers patterns outside the normal system behaviour. Within information security, this can be useful in detecting cyber-attacks and malicious actions.
Problem management
- The reason why organizations struggle with problem management is because there is an extensive amount of analysis that is required to identify the underlying causes of a problem. This practice will benefit from the emerging AIOps platforms with data collected from different sources.
- The main reason for adapting AIOps platforms is to enable the machine to see correlations and patterns in data, to identify problems, and perform automatic actions to avoid incidents.
AIOps used to support the business level
Today, almost anything can be monitored. When an organization develops competency and experience within AIOps it can also be utilized beyond IT operations. IT operations might first explore how AIOps can be used for all operational aspects of a service. The next step could be to provide business managers with real-time insights of the impact of IT on business, keeping them informed and enabling them to make decisions based on the relevant data.
With the functionality of an AIOps platform, IT operations could design dashboards with real-time information and analytics that really matters, both for operations and for the business.
As an example, many organisations of today are adopting the triple bottom line approach. This approach is referring to an accounting framework covering not only financial, but also social and environmental aspects. For an organisation it marks a shift away from short-term financial goals to long-term sustainability goals as an integrated method of doing business. A target like this will affect which data to measure. As an example, AI could be used to understand realtime customer satisfaction measures and how to react to it.
It could help analysing the general business health, as well as the organizations accumulated carbon footprint day by day. Ideas of more aspects that could be monitored are described in Table 4.1. More information about the triple bottom line approach can be found in the ITIL 4 publication Drive Stakeholder Value.
Profit | People | Planet | |
---|---|---|---|
Business Level | The status of an ordering process Sales and profit per hour and per day Real-time consumer knowledge (locations, purchase patterns) | Real-time customer satisfaction Contribution to local society | Automated process to minimize the global footprint (for example, avoid goods sent around the world, when local distribution is available, reduced production of clothes that add microplastic in the washing water, and reduced use of chemicals) |
IT | Overall availability Cost of downtime Solve problems/root causes Number of people operating the service | Employee satisfaction Number of routine tasks automated for people to be used on more important areas | Minimizing energy consumption in a data centre Effective utilization of servers Effective reuse or recycle of equipment |
Table 4.1 Using AIOps to measure business and IT targets with a triple bottom line mindset
For organizations to reach their goals, the first step is to start monitoring the correct things, then to present the data in a way that makes it is easier to make decisions. Some decisions might even be possible to automate.
SUMMARY OF THE DIFFERENT TYPES OF USE
Some AIOps functionality has been used by IT operations for a long time, while other areas are new and emerging. The biggest difference within the emerging functionality is the amount of data analysed and the scale of the use of automation and AI. Tables 4.2, 4.3, and 4.4 summarize different use of AI within IT operations.
AIOps example | Description | Current maturity | Value provided |
---|---|---|---|
Automation in general | Standardize and automate routine tasks. (script to request new virtual server, etc.) | Common Increased use | Save time Avoid human errors |
Automation at the service desk | Automate service requests, provide ordering forms and standard workflow. | Common Increased use | Customer satisfaction. Enable the consumer to help themselves as they need it. |
Table 4.2 Basic AIOps functionality in operations and service management
AiOps example | Description | Current maturity | Value provided |
---|---|---|---|
Cloud computing in general | Cloud computing represents an architectural shift in IT. Most cloud-based platforms have possibilities for a variety of AIOps functionality, designed to monitor, and manage tasks both for the provider and their customers. | The platform service provider uses of AIOps to manage their platforms | Elastic platforms with automatic resources allocation based on usage |
Cloud-based DevOps platforms | Cloud based DevOps platforms are optimized for DevOps. The platform teams create built-in AIOps functionality, designed to support the full development pipeline for a DevOps development team, enabling them to take full responsibility for their own value stream. Example of self help functionality:
| Common and crucial part of a DevOps platform | Makes it possible for the DevOps development team to be autonomous Short lead time |
Table 4.3 AIOps within cloud computing
AIOps examples | Description of AIOps Functionality | Current maturity | Value provided |
---|---|---|---|
Step 1: establish a big data AIOPS platform | Data collection from different sources Filtering out unimportant data correlations Recognize overall patterns Providing real-time reporting dashboards Using historical data to predict the future | Quite new Few vendors offer comprehensive, integrated AIOps platforms yet, but several provide solutions with basic functionality | Making sense of data Analyse connections Provide control Enable knowledge and understanding |
Step 2: Start using Machine Learning to aggregate, analyse and act | Algorithmic training on a large volume of data Anomaly detection, learn what is normal system behaviour, and react accordingly when it is not normal Isolate affected areas, establish timeline, and seek to identify root causes | Not yet very mature, but emerging As AIOps big data platform solutions mature, Gartner expect it to be | Less downtime Identify problems and their root causes, even before it becomes an incident |
Table 4.4 AIOps big data platforms
Before starting your AIOps journey
REMEMBER THE GUIDING PRINCIPLES
For any AIOps initiative the ITIL guiding principles will provide useful direction.
Focus on value
Start with AIOps where you need it most. Choose a pilot where AIOps can solve a real challenge and provide real value.Start where you are
Assess the current situation according to the area you have chosen as a pilot. What kind of available data can be utilized by AI tools? Is the data set big enough? Is it reliable?Progress iteratively with feedback
Operations teams adopting AI tools will need to explore and learn how to utilize AI and machine learning within operations. They could use AI tools that allow for insight, so they can understand and trust the algorithms used by the machine learning process.Collaborate and promote visibility
Data from different sources should be analysed together in search for high-level correlations and patterns.This will require collaboration and make the results visible for all.
Think and work holistically
The ITIL service value chain model can help identify the scope of an AIOps project. It is ideal to take a holistic view on how the services are delivered in order to understand how AIOps can assist in the value creation. Sub-optimization, which improves one part of the value chain, may not lead to better end results.Keep it simple and practical
Choose a specific case that is simple and practical where the operation team can build skills andexperience step by step. Then, the team will be prepared as the challenges grow and the AIOps tools become more developed.
Optimize and automate
Operation teams are normally tasked with time-consuming routine jobs. New possibilities within scripting use infrastructure as code and the key mindset of an operation team should be to ‘optimize it first, then automate everything you can’.THINK OF THE FOUR DIMENSIONS OF SERVICE MANAGEMENT
For every AIOps initiative, it can also be useful to assess the four dimensions of service management.
The Four Dimensions | Questions to ask before the AIOps Initiatives |
---|---|
Organizations and people | What are the goals and objectives? Who are the most important customers? How can their service needs be measured? What is the culture for continual improvement, standardization, and automation like in IT operation? What is the current knowledge and experience with AIOps? Does the organization have the necessary business understanding and analytical skills to utilize AIOps? |
Value streams and processes | What is the most important value stream? How can it be optimized? How can it be automated to avoid time consuming routine tasks? How can the important steps be monitored? How can issues be identified? |
Information and technology | What kind of monitoring is needed to support the different value streams? What kind of data would be useful? How can data be gathered? What kind of tools are needed, in order to store, process, and analyse a large volume of data? What kind of competency and technology is needed to be able to utilize the data for automatic decisions? |
Partners and suppliers | Who are the vendors that support our need? What area of expertise does the supplier need to have? |
Table 5.1 How the four dimensions of the service management should be taken in consideration
More information on the guiding principles and four dimensions of service management can be found in the ITIL Foundation: ITIL 4 Edition publication.
Conclusion
Undoubtedly, AIOps will change the way IT services are managed in the future. AIOps can be used in a wide range of areas within IT operations and contributes to a high number of innovations. The real value will come with the concurrent use of multiple data sources, analysed together to provide real-time insight both for IT and for the organization.There are several reasons of the breakthrough in AI and machine learning right now; the key enablers are:
- improved algorithms
- increased processing capabilities of the computers
- the ability to use the algorithms on large volumes of quality data.
AIOps will be an important part of service management in the future. It will require new knowledge and skills within an IT operations team, to really understand what AI and machine learning can do, both the possibilities and the limits. Therefore, organizations should start building the necessary abilities now, to utilize the new technology as it becomes more mature.
End notes
How to get started with AIOps.
Available at: https://www.gartner.com/smarterwithgartner/how-to-get-started-with-aiops/ [Accessed 19 December 2019]
About the author
Signe-Marie Hernes Bjerke has an M.Sc. of Informatics from the University of Oslo and has more than 20 years of experience within IT from a wide range of IT service providers. For 16 years she has been working for DNV GL in Norway, helping customers with process improvement, quality assurance, risk management, and continual improvement, both within IT and on the business side, and the integration between business and IT. In 2017 she decided to start as a freelancer in her own company Teambyggerne AS.
Signe-Marie is an expert in IT Service Management and ITIL, a best practice framework providing a process-oriented approach towards high quality services. She is certified tutor both on ITIL Foundation and Expert level, and has been Senior Examiner for ISEB, APMG and Axelos. Signe-Marie was part of the group founding itSMF Norway in 2003 and has been in the board form 2003 until 2015, the last two years as Chairman. She was the Norwegian member of the ITIL Advisory Group during the development of ITIL v3 and has now been part of the authoring team for the AXELOS ITIL 4 publication Drive Stakeholder Value.